Research
I am interested in 3D computer vision, machine learning, and robotics. My research goal is
to build intelligent systems which are able to achieve an effective and efficient perception
and understanding of 3D scenes. In particular, my research focuses on large-scale point
cloud segmentation, dynamic point cloud processing, point cloud tracking, and local surface
matching. If you are interested in my research or have any use cases that you want to share,
feel free to contact me!
Skills:
Python |
Tensorflow |
PyTorch |
ROS |
Published Venues:
CVPR(7) ✔
IEEE TPAMI(4) ✔
ECCV(3) ✔
IJCV(1) ✔
NeurIPS(1) ✔
|
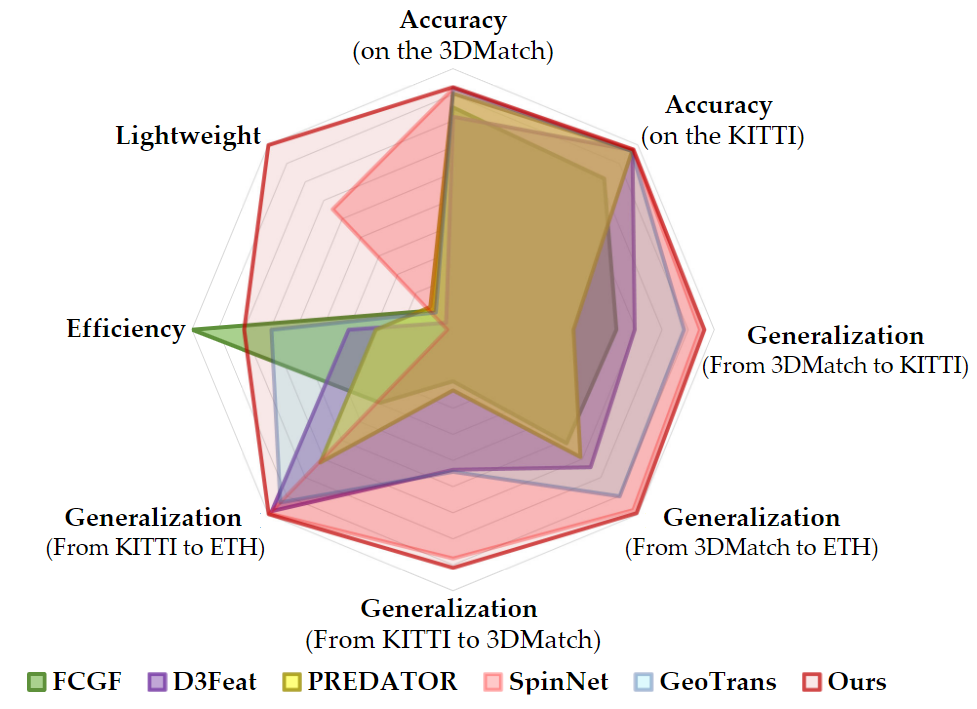 |
BUFFER: Balancing Accuracy, Efficiency, and Generalizability in Point Cloud Registration
S. Ao, Q. Hu, H. Wang, K. Xu, Y. Guo
CVPR 2023
Paper /
Code
The paper proposes a point cloud registration method called "BUFFER" that balances accuracy, efficiency, and generalizability. The method combines point-wise and patch-wise techniques to overcome their inherent drawbacks and achieve superior performance in all three aspects. The method's components have been carefully crafted to tackle specific issues, resulting in better registration success rates and faster processing times compared to existing methods. Extensive experiments on real-world scenarios validate the effectiveness of the proposed method.
|
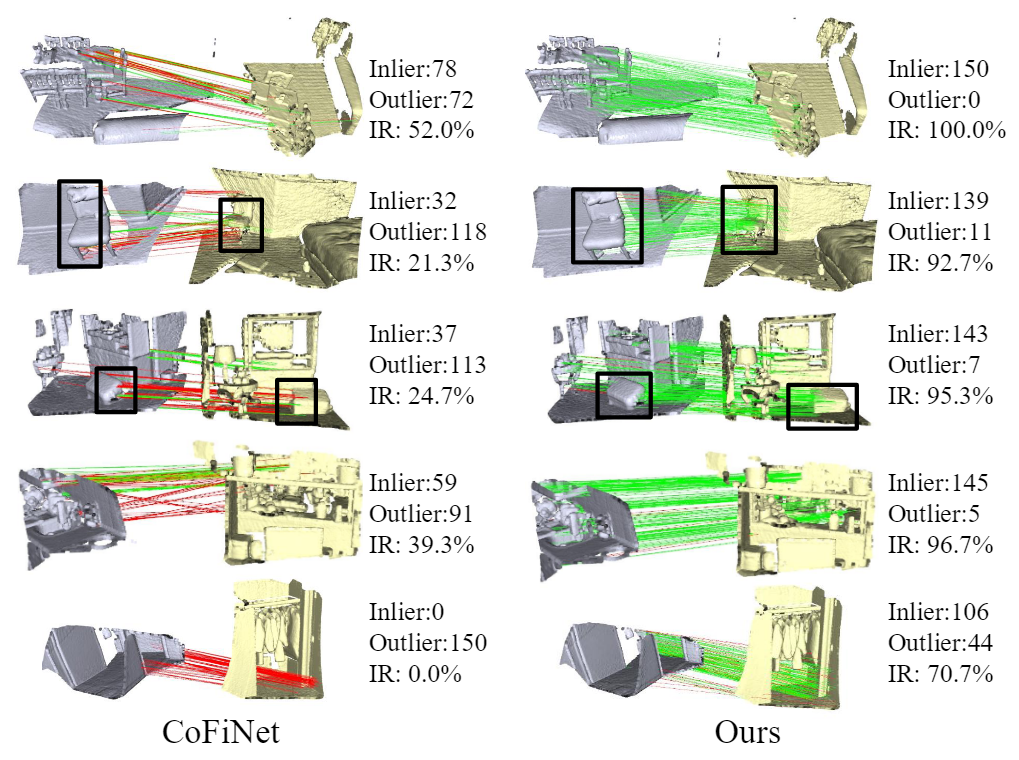 |
RoReg: Pairwise Point Cloud Registration with Oriented Descriptors and Local Rotations
H. Wang, Y. Liu, Q. Hu, B. Wang, J. Chen, Z. Dong, Y. Guo, W. Wang, B. Yang
IEEE Transactions on Pattern Analysis and Machine Intelligence,
2023 (IF=24.31)
Paper /
Code
RoReg is a point cloud registration framework that utilizes oriented descriptors and estimated local rotations to improve the feature description, feature detection, feature matching, and transformation estimation stages of the registration pipeline. The proposed RoReg-Desc descriptor is used to estimate local rotations, enabling the development of a rotation-guided detector, a rotation coherence matcher, and a one-shot-estimation RANSAC, all of which enhance the registration performance. RoReg achieves state-of-the-art results on popular datasets, including 3DMatch and 3DLoMatch, and also generalizes well to the outdoor ETH dataset. .
|
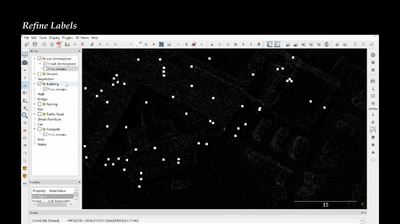 |
SQN: Weakly-Supervised Semantic Segmentation of Large-Scale 3D Point Clouds
with 1000× Fewer Labels
Q. Hu, B. Yang, G. Fang, A. Leornadis, Y. Guo, N. Trigoni, A.
Markham
ECCV, 2022
Paper /
Demo /
Annotation /
Code
We propose a new weak supervision method to
implicitly augment the total amount of available supervision signals, by leveraging the
semantic similarity between neighboring points. Extensive experiments demonstrate that
the proposed Semantic Query Net- work (SQN) achieves state-of-the-art performance on six large-scale open datasets under weak supervision schemes, while requiring only 1‰ labeled points for training.
|
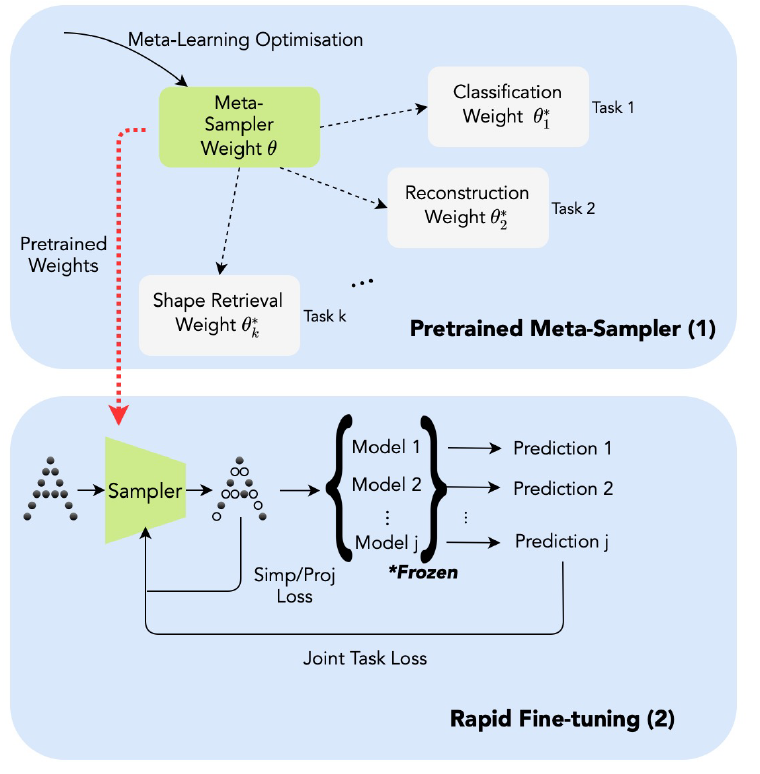 |
Meta-Sampler: Almost-Universal yet Task-Oriented Sampling for Point Clouds
T. Cheng, Q. Hu*, Q. Xie, N. Trigoni, A. Markham
(*indicates corresponding author)
ECCV 2022
Paper /
Code
Sampling is a key operation in point-cloud task and acts to increase computational efficiency and tractability by discarding redundant points. Universal sampling algorithms (e.g., Farthest Point Sampling) work without modification across different tasks, models, and datasets, but by their very nature are agnostic about the downstream task/model. We propose an almost-universal sampler, in our quest for a sampler that can learn to preserve the most useful points for a particular task, yet be inexpensive to adapt to different tasks, models, or datasets.
|
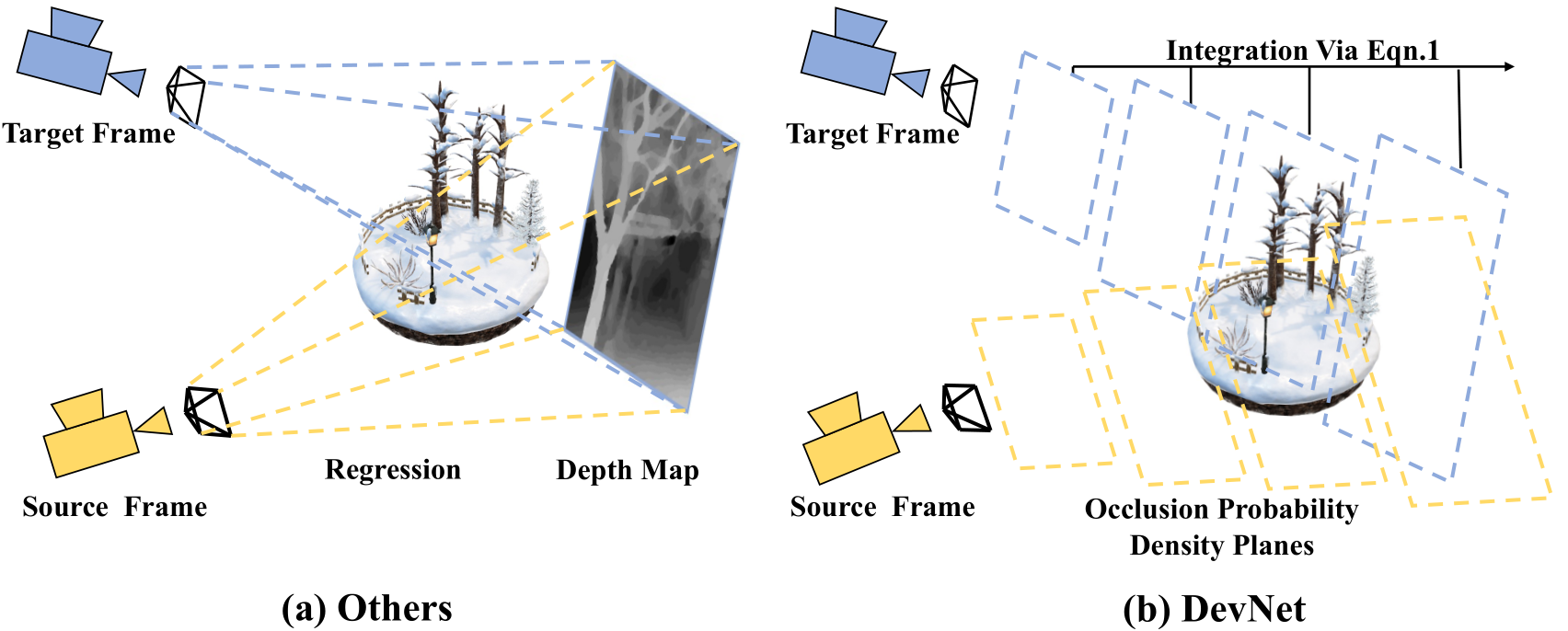 |
DevNet: Self-supervised Monocular Depth Learning via Density Volume Construction
K. Zhou, L. Hong, C. Chen, H. Xu, C. Ye, Q. Hu, Z. Li
ECCV 2022
Paper /
Code
Self-supervised depth learning from monocular images normally relies on the 2D pixel-wise photometric relation between temporally adjacent image frames. However, they neither fully exploit the 3D point-wise geometric correspondences, nor effectively tackle the ambiguities in the photometric warping caused by occlusions or illumination inconsistency. To address these problems, this work proposes Density Volume Construction Network (DevNet), a novel self-supervised monocular depth learning framework, that can consider 3D spatial information, and exploit stronger geometric constraints among adjacent camera frustums.
|
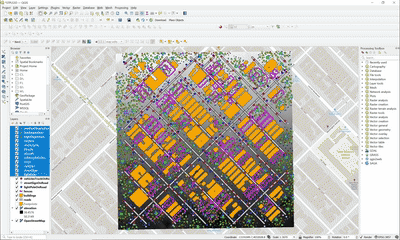 |
STPLS3D: A Large-Scale Synthetic and Real Aerial Photogrammetry 3D Point Cloud Dataset
M. Chen, Q. Hu*, T. Hugues, A. Feng, Y. Hou, K. McCullough, L. Soibelman
(*indicates corresponding author)
BMVC 2022
Paper/
Demo /
Project Page /
Code
We explore the procedurally synthetic 3D data generation paradigm to equip individuals with the full capability of creating large-scale annotated photogrammetry point clouds. Specifically, we introduce a synthetic aerial photogrammetry point clouds generation pipeline that takes full advantage of open geospatial data sources and off-the-shelf commercial packages.
|
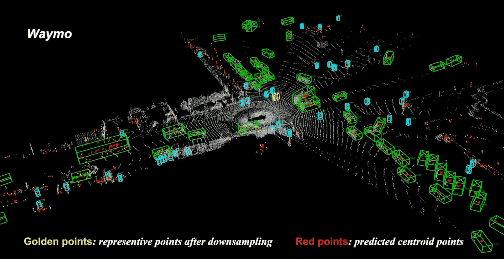 |
Not All Points Are Equal: Learning Highly Efficient Point-based Detectors for 3D LiDAR Point Clouds
Y. Zhang, Q. Hu*, G. Xu, Y. Ma, J. Wan, Y. Guo
(*indicates corresponding author)
CVPR 2022 (Oral)
Paper/
Demo /
Code
We study the problem of efficient object detection of 3D LiDAR point clouds. In this paper, we propose a highly-efficient single-stage point-based 3D detector in this paper, termed IA-SSD, based on the fact that foreground points are inherently more important than background points for object detectors.
|
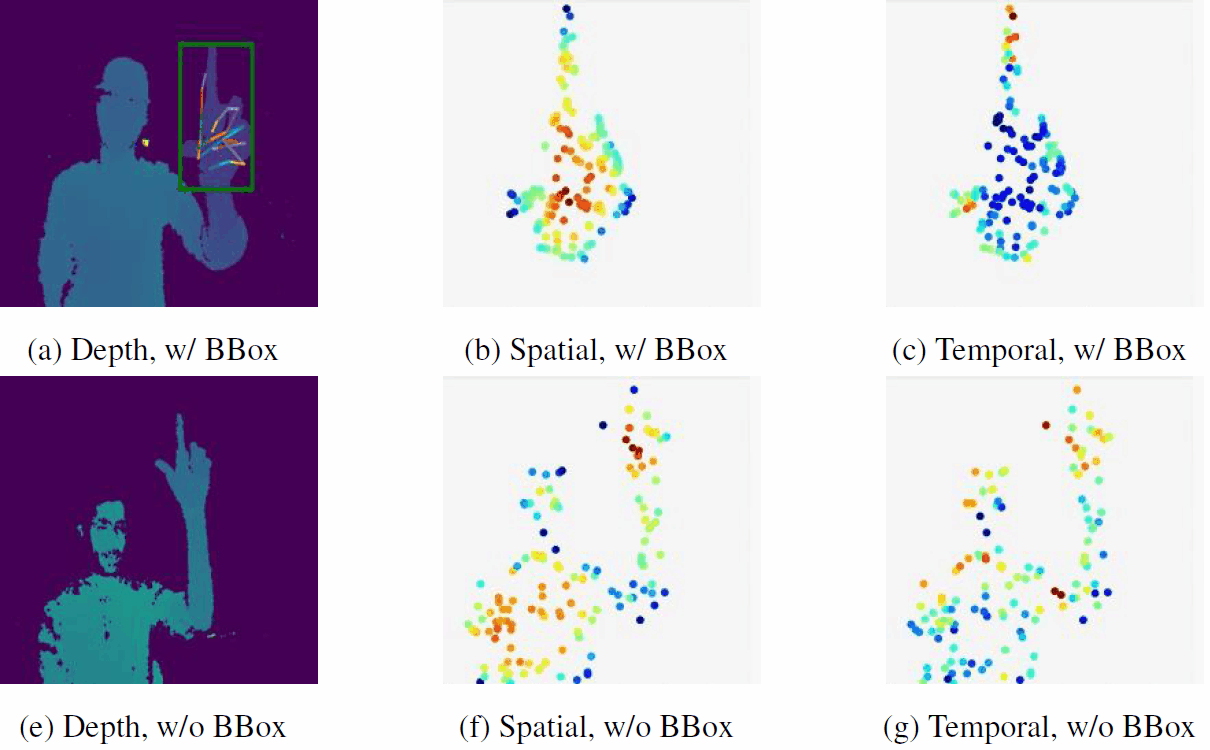 |
No Pain, Big Gain: Classify Dynamic Point Cloud Sequences with Static Models by Fitting Feature-level Space-time Surfaces
J. Zhong, K. Zhou, Q. Hu*, B. Wang, N. Trigoni, A. Markham
(*indicates corresponding author)
CVPR 2022
Paper/
Code
Scene flow is a powerful tool for capturing the motion field of 3D point clouds. To capture 3D motions without explicitly tracking correspondences, we propose a kinematics-inspired neural network (Kinet) by generalizing the kinematic concept of ST-surfaces to the feature space.
|
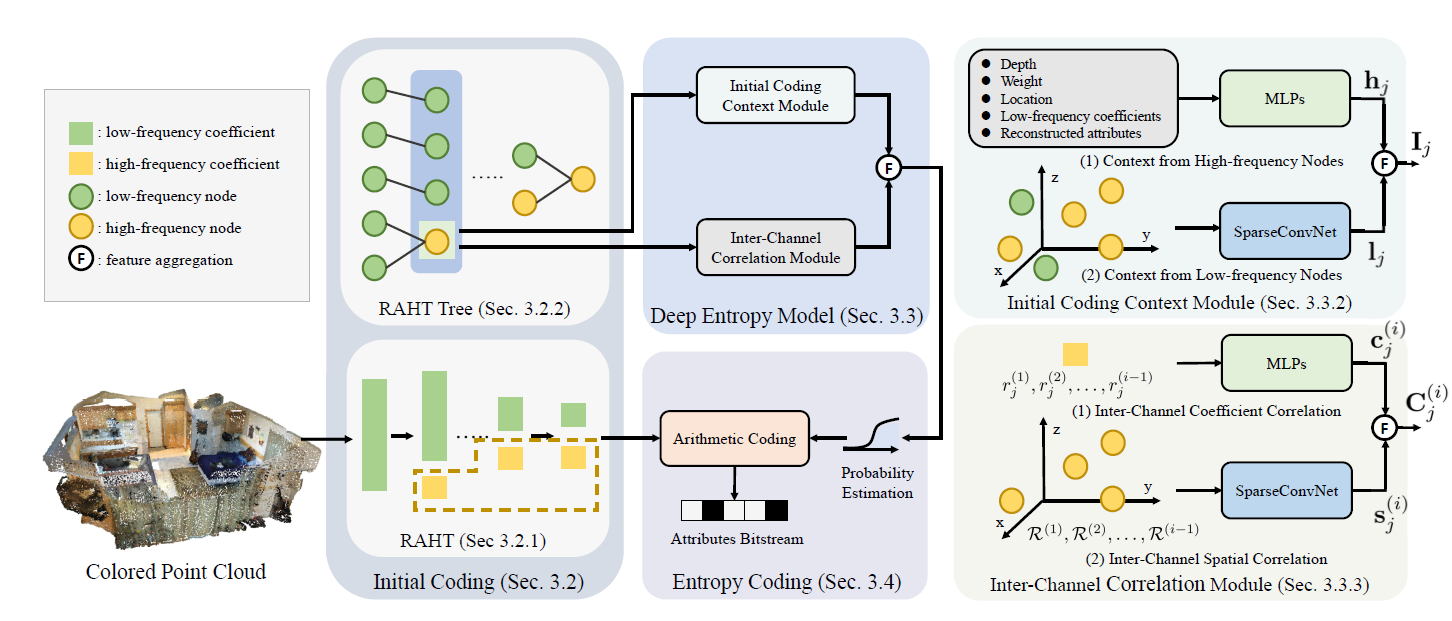 |
3DAC: Learning Attribute Compression for Point Clouds
G. Fang, Q. Hu, H. Wang, Y. Xu, Y. Guo
CVPR 2022
Paper
We study the problem of attribute compression for large-scale unstructured 3D point clouds. Through an in-depth exploration of the relationships between different encoding steps and different attribute channels, we introduce a deep compression network, termed 3DAC, to explicitly compress the attributes of 3D point clouds and reduce storage usage in this paper.
|
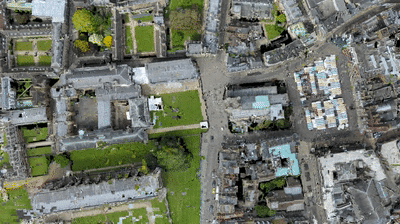 |
SensatUrban: Learning Semantics from Urban-Scale Photogrammetric Point Clouds
Q. Hu, B. Yang, S. Khalid, W. Xiao, N. Trigoni, A.
Markham
International Journal of Computer Vision,
2022 (IF=11.54)
Paper /
Demo /
Code
This is a journal extension of the SensatUbran dataset, with more extensive experiments on the cross-dataset generalization, semantic learning with fewer labels and self-supervised pre-training. We provide more details regarding the data acquisition and semantic annotation.
|
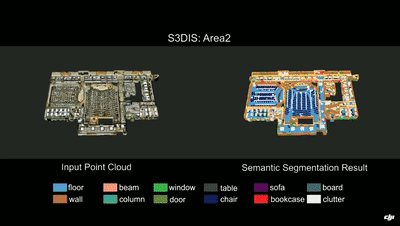 |
Learning Semantic Segmentation of Large-Scale Point Clouds with Random Sampling
Q. Hu, B. Yang, L. Xie, S. Rosa, Y. Guo, Z. Wang,
N. Trigoni, A. Markham
IEEE Transactions on Pattern Analysis and Machine Intelligence,
2021 (IF=24.31)
Paper /
Demo /
Code
This is a journal extension of the RandLA-Net, with more extensive experiments on the Toronto3D, NPM3D, ScanNet and DALES. We provide more details
regarding the entire architecture and the implementation of our RandLA-Net, including all
specifics of encoding/decoding neural layers and particulars of the training strategy.
|
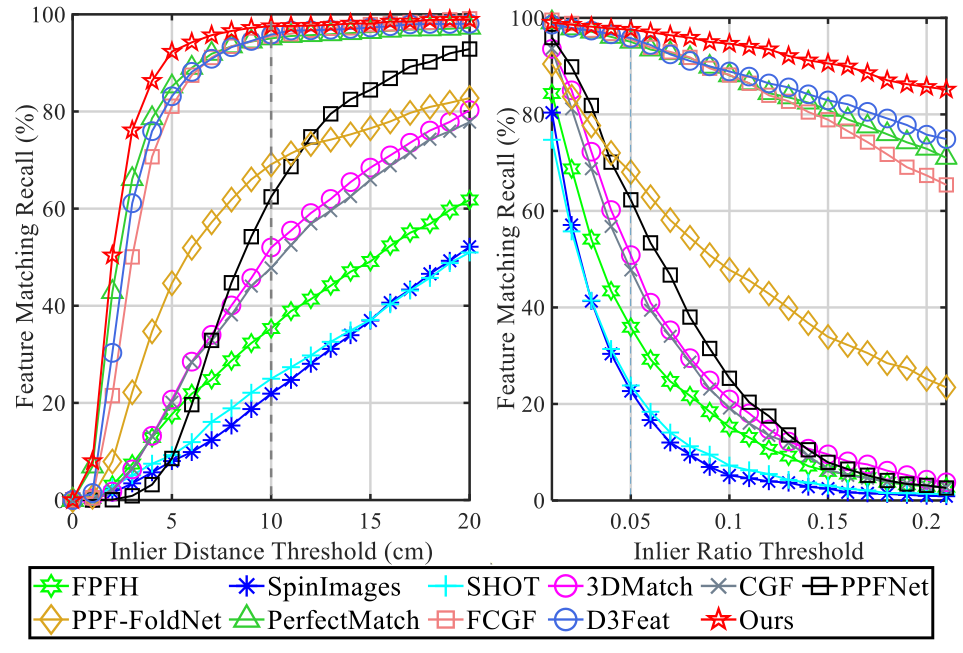 |
SpinNet: Learning a General Surface Descriptor for 3D Point Cloud
Registration
S. Ao*, Q. Hu*, B. Yang, A. Markham, Y. Guo
CVPR, 2021
(* indicates equal contribution)
arXiv /
Demo /
Project Page /
Code
We introduce a new, yet conceptually simple,neural architecture to extract local features which are rotationally invariant whilst sufficiently informative to enable accurate registration. A Spatial Point Transformer is first introduced to map the input local surface into a carefully designed cylindrical space, enabling end-to-end optimization with SO(2) equivariant representation. A Neural Feature Extractor which leverages the powerful point-based and 3D cylindrical convolutional neural layers is then utilized to derive a compact and representative descriptor for matching.
|
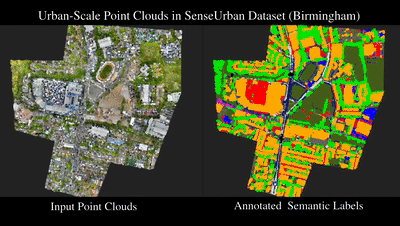 |
Towards Semantic Segmentation of Urban-Scale 3D Point Clouds: A
Dataset, Benchmarks and Challenges
Q. Hu, B. Yang, S. Khalid, W. Xiao, N. Trigoni, A.
Markham
CVPR, 2021
arXiv /
Demo /
Project Page /
Code
We introduce an urban-scale
photogrammetric point cloud dataset with nearly three billion richly annotated points, which is five times the number of labeled points than the existing largest point cloud dataset. We extensively evaluate the performance of state-of-the-art algorithms on our dataset and provide a comprehensive analysis of the results. In particular, we identify several key challenges towards urban-scale point cloud understanding.
|
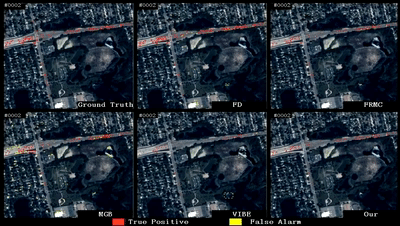 |
Detecting and Tracking Small and Dense Moving Objects in Satellite Videos: A Benchmark
Q. Yin, Q. Hu*, H. Liu, F. Zhang, Y. Wang, Z. Lin, W. An, Y. Guo
(*indicates equal contribution)
IEEE Transactions on Geoscience and Remote Sensing (IF=8.125)
Paper/
Demo /
Project Page /
Code
In this paper, we first build a large-scale satellite video dataset with rich annotations for the task of moving object detection and tracking. This dataset is collected by the Jilin-1 satellite constellation and composed of 47 high-quality videos with 1,646,038 instances of interest for object detection and 3,711 trajectories for object tracking. We
|
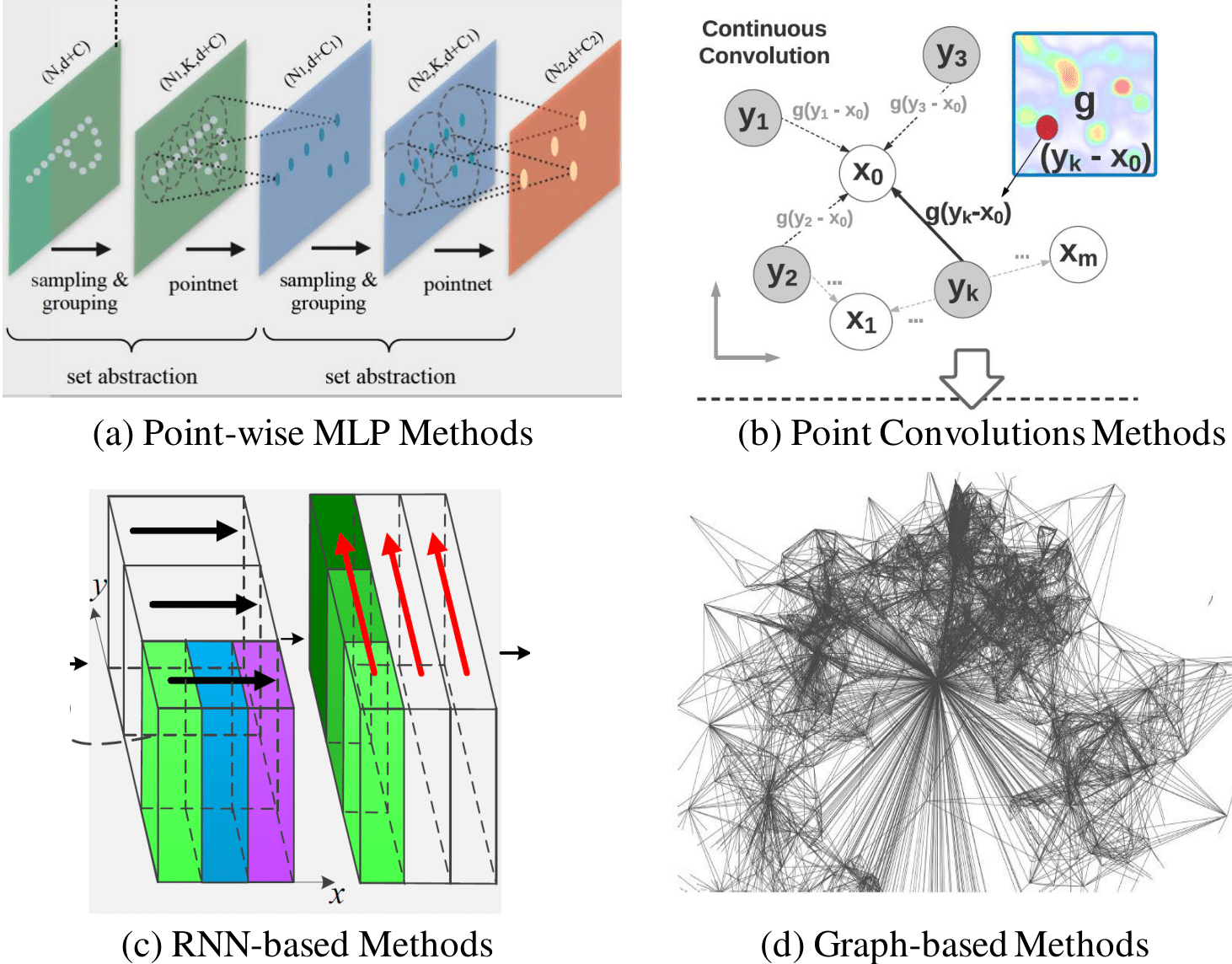 |
Deep Learning for 3D Point Clouds: A Survey
Y. Guo*, H. Wang*, Q. Hu*, H. Liu*, L. Liu, M.
Bennamoun
IEEE Transactions on Pattern Analysis and Machine Intelligence,
2020 (IF=24.31)
(* indicates equal contribution)
arXiv /
bibtex
/
News:
(专知,
CVer) /
Project
page
We presents a comprehensive review of recent progress in deep learning methods for point clouds. It covers three major tasks, including 3D shape classification, 3D object detection, and 3D point cloud segmentation. It also presents comparative results on several publicly available datasets, together with insightful observations and inspiring future research directions.
|
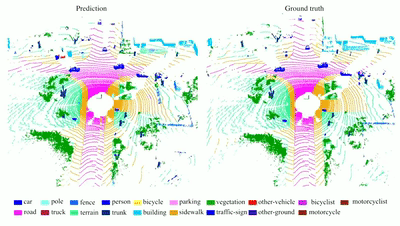 |
RandLA-Net: Efficient Semantic Segmentation of Large-Scale
Point
Clouds
Q. Hu, B. Yang, L. Xie, S. Rosa, Y. Guo, Z. Wang,
N. Trigoni, A. Markham
Computer Vision and Pattern Recoginition (CVPR), 2020 (Oral, 335/6656)
arXiv /
Demo /
News:
(新智元,
极市平台,
AI科技评论)
/
Code
We propose a simple and efficient
neural architecture for 3D semantic segmentation on large-scale point
clouds. It achieves the SOTA performance on Semantic3D and SemanticKITTI
(Nov 2019), with up to 200x fast than existing approaches.
|
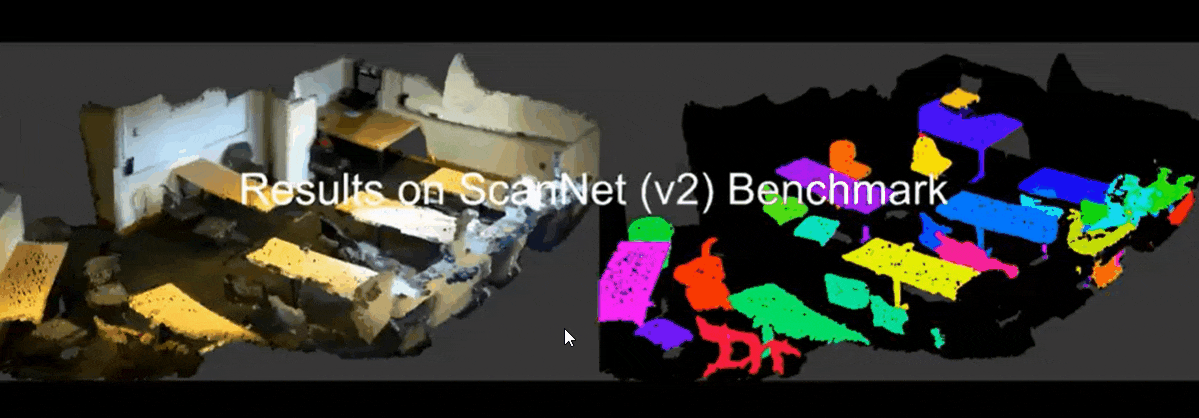 |
Learning Object Bounding Boxes for 3D Instance
Segmentation
on Point Clouds
B. Yang, J. Wang, R. Clark, Q. Hu, S. Wang, A.
Markham,
N.
Trigoni
Neural Information Processing Systems (NeurIPS), 2019 (Spotlight, 200/6743)
arXiv/
Reddit/
Demo/
News:
(新智元,
将门创投,
泡泡机器人)/
Code
We propose a simple and efficient neural architecture for accurate 3D instance segmentation on point clouds. It achieves the SOTA performance on ScanNet and S3DIS (June 2019).
|
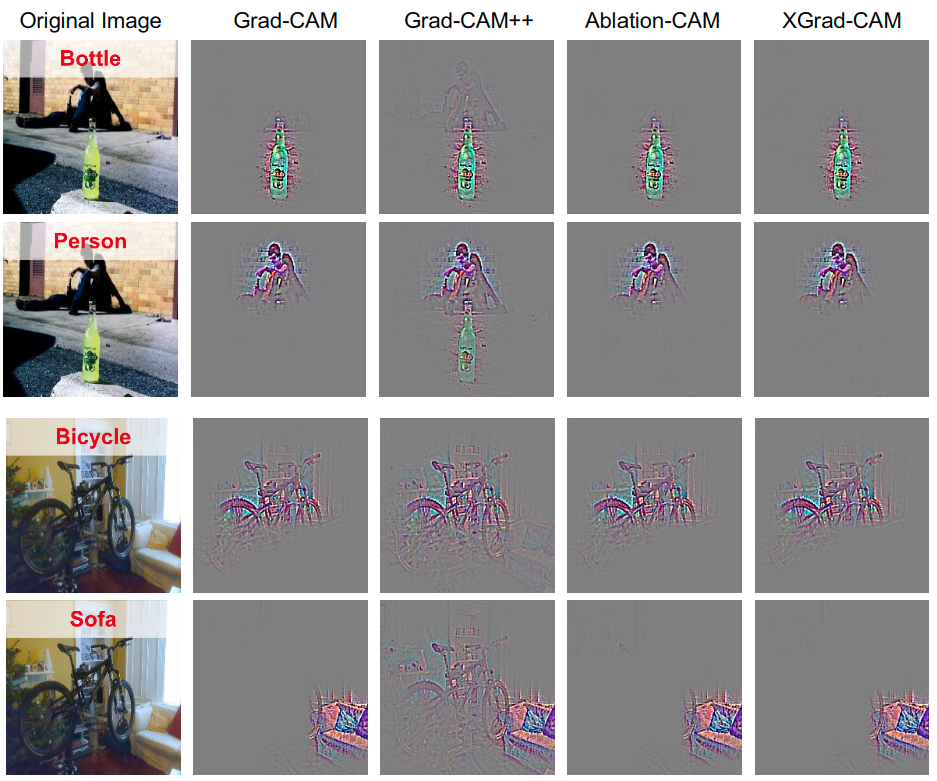 |
Axiom-based Grad-CAM: Towards Accurate Visualization
and
Explanation
of CNNs
R. Fu, Q. Hu, X. Dong, Y. Guo, Y. Gao, B.
Li
British Machine Vision Conference (BMVC), 2020 (Oral, 30/670)
arXiv/
Code
We introduce two axioms
--Conservation and Sensitivity -- to the visualization paradigm of the CAM methods. Meanwhile, a dedicated Axiom-based Grad-CAM (XGrad-CAM) is proposed to satisfy these axioms as much as possible. Experiments demonstrate that XGrad-CAM is able to achieve better visualization performance than Grad-CAM, while also be class-discriminative and easy-to-implement compared with Grad-CAM++ and Ablation-CAM.
|
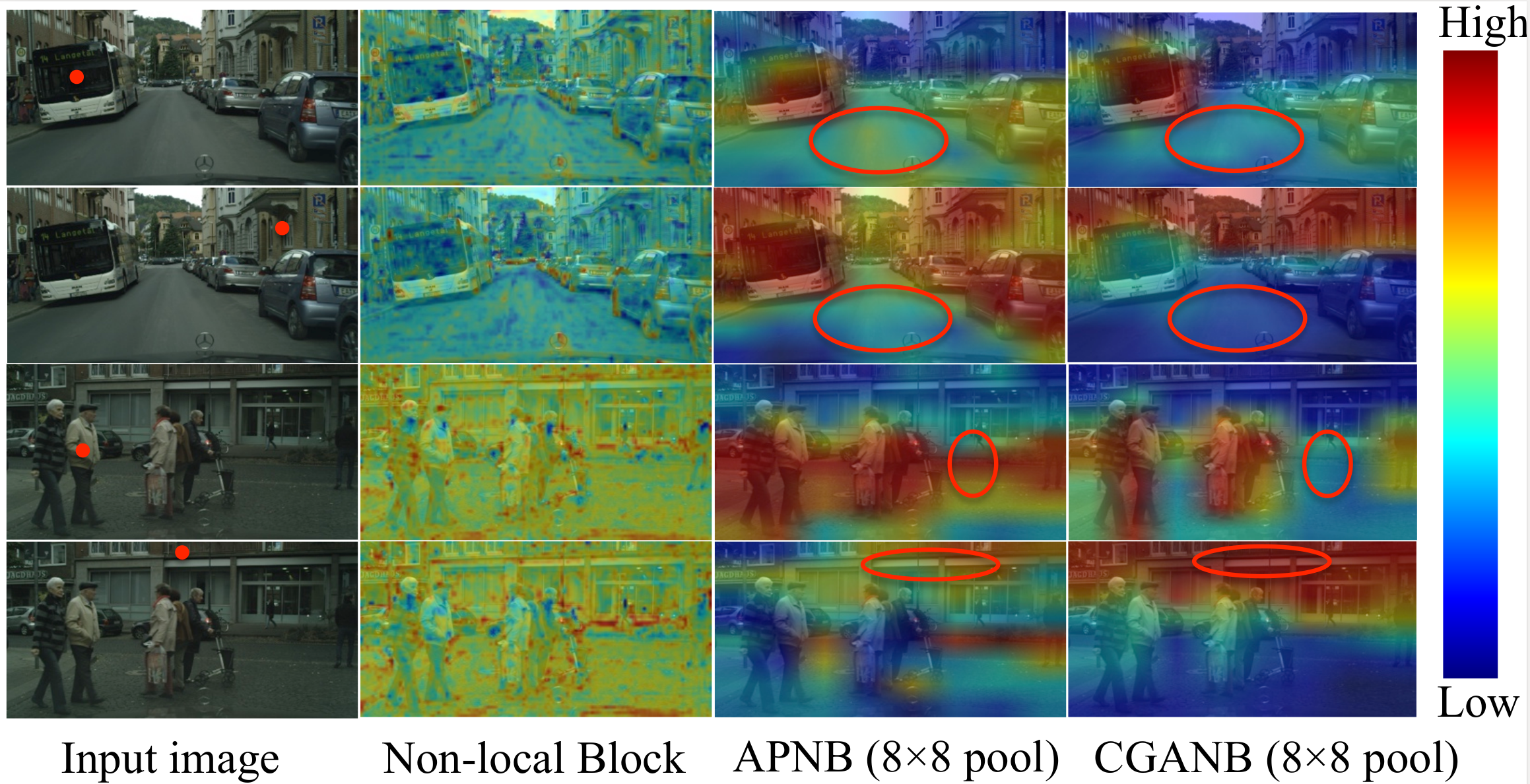 |
CGAN-Net: Class-Guided Asymmetric Non-local
Network
for
Real-time Semantic Segmentation
H. Chen, Q. Hu, J. Yang, J. Wu, Y.
Guo
International Conference on Acoustics, Speech, & Signal
Processing (ICASSP), 2021
we introduce a
Class-Guided Asymmetric Non-local Network (CGAN-Net) to
enhance the class-discriminability in learned feature map,
while
maintaining real-time efficiency. The key to our approach is
to
calculate the dense similarity matrix in coarse semantic
prediction
maps, instead of the high-dimensional latent feature
map.
|
|
Robust Long-term Tracking via Instance Specific
Proposals
H. Liu, Q. Hu, B. Li, Y. Guo
IEEE Transactions on Instrumentation and Measurement (IEEE
TIM),
2019
(IF=5.332)
We propose an efficient and robust tracker for long-term object tracking, which is based on instance specific proposals. In particular, an instance-specific proposal generator is embedded into the error correction module to recover lost target from tracking failures.
|
|
Semi-Online Multiple Object Tracking Using Graphical
Tracklet
Association
J. Wang, Y. Guo, X. Tang, Q. Hu, W. An
IEEE Signal Processing Letters (IEEE SPL), 2018
(IF=3.27)
Demo
We propose a semi-online
MOT method using online discriminative appearance learning and tracklet association with a sliding window. We connect similar detections of neighboring frames in a temporal window, and improve the performance of appearance feature by online discriminative appearance learning. Then, tracklet association is performed by minimizing a subgraph decomposition cost.
|
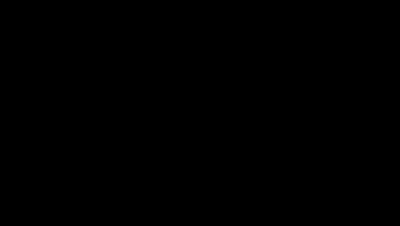 |
Object tracking using multiple features and adaptive
model
updating
Q. Hu, Y. Guo, Z. Lin, W. An, H. Cheng
IEEE Transactions on Instrumentation and Measurement (IEEE
TIM),
2018
(IF=5.332)
We proposea to combine a
2-D location filter with a 1-D scale filter to jointly estimate the state of object under tracking, and three complementary features are integrated to further enhance the overall tracking performance. A penalty factor is also defined to achieve a balance between stability and flexibility, especially when the object is under occlusion.
|
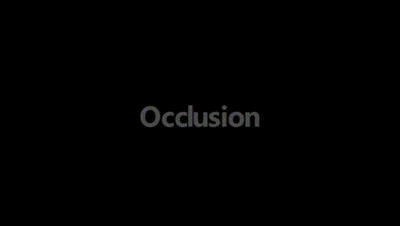 |
Long-term Object Tracking with Instance Specific
Proposals
H. Liu, Q. Hu, B. Li, Y. Guo
24th International Conference on Pattern Recognition
(ICPR),
2018
We propose a tracker named Complementary Learners with Instance-specific Proposals (CLIP). The CLIP tracker consists of a translation filter, a scale filter, and an error correction module. The error correction module is activated to correct the localization error by an instance-specific proposal generator, especially when the target suffers from dramatic appearance changes.
|
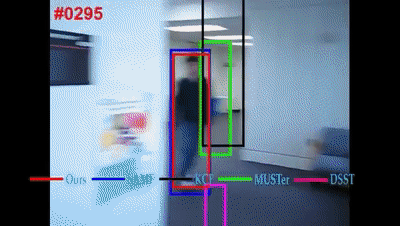 |
Correlation Filter Tracking: Beyond an Open-loop
System
Q. Hu, Y. Guo, Y. Chen, J. Xiao, W. An
British Machine Vision Conference (BMVC), 2017
Demo /
bibtex
/
Code
We interpret object
tracking as a closed-loop tracking problem, and add a feedback loop to the tracking process by introducing an efficient method to estimate the localization error. We propose a generic self-correction mechanism for CF based trackers by introducing a closed-loop feedback technique.
|
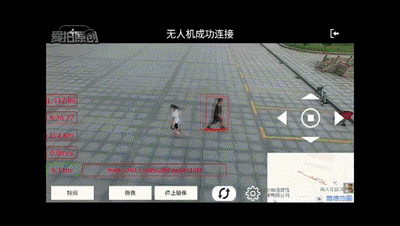 |
Robust and real-time object tracking using
scale-adaptive
correlation
filters
Q. Hu, Y. Guo, Z. Lin, X. Deng, W. An
Digital Image Computing: Techniques and Applications
(DICTA), 2016 (Oral)
We represent the target in kernel feature space and train a classifier on a scale pyramid to achieve adaptive scale estimation. We then integrate three complementary features to further enhance the overall tracking performance.
|
|